The extent of migration among populations drives population structure. With enough migration, populations become homogeneous and behave as a single larger population. As migration rates decrease, populations drift apart and become differentiated. By measuring the amount of differentiation, we can determine the extent of migration between them. But what happens when there are unsampled populations also exchanging migrants?
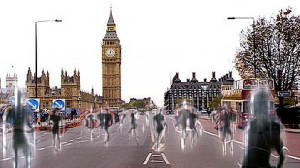
Ghosts are populations that represent the collective effect of unsampled populations on estimates of migration rates among populations sampled. A ghost can be a single population from a neighboring site that exchanges migrants with the sampled populations, it can be a collection of populations surrounding the sampled populations, it can be genetic diversity within the sampled populations that went unsampled, or it can be the total genetic diversity of a larger population from which the sampled populations are a subset. Simply put, a ghost is any unsampled population that is connected by migration to the sampled populations. Especially in marine ecosystems, it’s almost impossible to sample all populations, so there will always be unsampled ghost populations.
How much effect do these ghost populations really have on estimates of migration rate?
Beerli (2004) tested the effects of ghost populations in coalescent estimates of migration rate (the term ‘ghost population’ was also coined by Beerli). Not surprisingly, he determined that the effect of ghosts on migration rate was dependent on the amount of migration from the ghosts into the sampled population. Only in cases where migration from the ghost population to the sampled populations was exceptionally high did the ghosts significantly affect the migration rate. Specific cases involve source/sink relationships where the ghost population is the source of genetic diversity and instances where migration between all populations and the ghost are large and symmetric. In these cases, genes from the ghost populations effectively swamp out local differentiation and you’re left measuring either just the ghost or the total diversity as a single population.
In general, when only two populations are sampled and the ghost ignored, effective size and migration rate are overestimated. This effect can be mitigated by ensuring that the dominant population (the population responsible for most of the genetic diversity) is sampled.
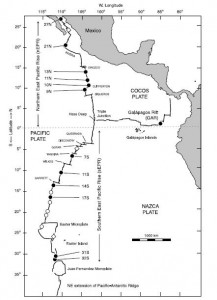
Slatkin (2005) went on to further define the effects of ghost populations. Through a series of coalescent-based models, he determined that there is no general solution to measuring the effects of ghost populations because the underlying processes will be different depending on which populations are sampled. It may be impossible to quantify the effects of ghost populations without knowing something about them.
Knowing this, how do we deal with ghost populations in real-world scenarios?
Audzijonytė and Vrijenhoek (2010) analyzed several data sets from deep-sea hydrothermal vents in the eastern Pacific to determine if gaps in sampling regimes really do affect migration between sampled sites. Unlike many marine systems, hydrothermal vents on mid-ocean ridges are distributed in a linear pattern that resembles a 1 dimensional stepping stone model. Despite their clean, linear distribution, there are few indications of isolation-by-distance among vent fauna and large genetic breaks that are consistent across many taxa. Most of these breaks correspond to geologic or oceanographic features, but one break – a decrease in migration across the equator – corresponds to an 1800 km sampling gap.
Do sampling gaps account for apparent population structure in linearly distributed hydrothermal vents?
Audzijonytė and Vrijenhoek (2010) used real-world data to model gene flow in a linear stepping stone and isolation-by-distance model to test the statistical power of sampling and whether or not barriers are real. By analyzing multiple taxa across the same geographic area, they determined that, in most cases, genetic breaks that corresponded with physical barriers were supported, but it is possible that gaps in the sampling regime could account for failure to detect isolation by distance.
So what does this all mean for aspiring population geneticists? For starters, it means that all populations behave differently, and you need to be aware of the assumptions you make before diving in to robust analyses. Dominant unsampled populations, or unsampled populations you suspect may have high migration into your system, will flood your sampled sites and bias results. But it many cases, a robust and comprehensive sampling scheme should be sufficient to mitigate the bias caused by ghost populations.
~Southern Fried Scientist
This post is part of the ongoing Crowdsourcing ConGen project. The goal of which is to produce a comprehensive and accessible introduction to Conservation Genetics for managers, conservationists, and interested parties that do not possess a technical background in genetics. Because of this, the focus of this piece is not on how to produce data or calculate each of the values discussed, but to provide the tools to understand and discuss assessments of population size and to be aware of the limitations of each technique.
As always, critical review of both the content and style is not only welcome, but essential for the success of this project. Anyone interested in digging deeper into the concepts presented here should peruse the following papers.
Audzijonytė, A., & Vrijenhoek, R. (2010). WHEN GAPS REALLY ARE GAPS: STATISTICAL PHYLOGEOGRAPHY OF HYDROTHERMAL VENT INVERTEBRATES Evolution DOI: 10.1111/j.1558-5646.2010.00987.x
Beerli, P. (2004). Effect of unsampled populations on the estimation of population sizes and migration rates between sampled populations Molecular Ecology, 13 (4), 827-836 DOI: 10.1111/j.1365-294X.2004.02101.x
SLATKIN, M. (2005). Seeing ghosts: the effect of unsampled populations on migration rates estimated for sampled populations Molecular Ecology, 14 (1), 67-73 DOI: 10.1111/j.1365-294X.2004.02393.x
ė
Oh wow. I know Asta Audzijonyte – she did her PhD in Helsinki. You’ve spelled her name wrong: there should be a dot over the e, IIRC.
And please don’t ask me how to pronounce her surname.
Fixed, thanks.
Ha! Yay!
BTW, Asta has had a past as an international mysid smuggler.