The focus on community and informal rules that were found to frequently structure successful commons management made apparent that the word ‘policy’ needed to be expanded. Policy scholars now look beyond the official written laws, reports, and regulations that are often written by a central government to multi-scalar rules that govern the structure and behavior in a system (Ostrom 2005). They include non-written cultural norms, religious prescriptions, and community ethics that are often more strongly followed that written, formal rules (Berkes 2008). Along with a more broadly defined concept of policy, commons scholars also introduced a more broad definition of institution within which these policies are made and enforced. Much like policies, institutions can be governmental as well as religious, moral, or cultural. Hanna and Jentoft (1996) give an appropriately broad definition for natural resource institutions: “institutions represent the arrangements which people devise to control their use of the natural environment”. Ostrom (2005) promotes an institutional analysis in order to encompass these new conceptions of policy and institution. Her work recently earned the Nobel prize and is rapidly becoming the most used framework for policy analysis.
Ostrom’s Institutional Analysis and Design (IAD) Framework is not specific for natural resources or even commons problems, but serves as a place to begin policy analysis for all sorts of policy problems, including education, gender relations, crime, and natural resources. A short description here does not fully describe the IAD framework – for that, refer to Ostrom’s 2005 book. To highlight a few pertinent features, the framework is centered around a decision-making arena called the action arena rather than on the formation of a particular policy. Inputs to the action arena include exogenous variables describing the biophysical characteristics, community factors, and existing rules relating the action arena in play. This action arena then interacts with action arenas at other levels of governance (operational, collective choice, constitutional, and super-constitutional) to form an outcome. The outcome is evaluated by some defined set of criteria and the process feeds back to become iterative. The main benefit of the framework is to allow for comparative studies between empirical studies by scholars around the world in many disciplines.
Particularly relating to natural resource management, Ostrom has offered a modified IAD framework for study of socioecological systems since many socioecological systems scholars have found the framework useful to their studies (Ostrom 2009). She states “a core challenge in diagnosing why some socioecological systems are sustainable whereas others collapse is the identification and analysis of relationships among multiple levels of these complex systems at different spatial and temporal scales”. The framework she offers is a means to organize complexity into a heuristic for standardized analysis. She states that complexity has four properties: nonlinearity, flows, diversity, and aggregation (Ostrom 1999). Each of these properties can be modeled within the IAD framework. Institutions prove useful as a unit of analysis because they define the ways in which humans and their environment interact (Adger 2000).
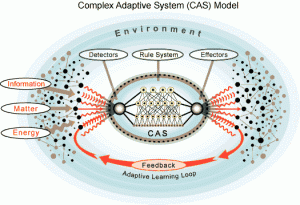
Complexity is another interdisciplinary concept that has moved into policy analysis through socioecological systems research. Socioecological systems are a type of complex adaptive system. The IAD framework offers a means accommodate that complexity to account both for multiscalar and multicausal interactions as well as feedbacks that lead to learning and adaptation. As a result of institutional analyses, policies are no longer seen as static entities but instead part of a larger system with the capability to learn and adapt. For a tangible example, McCay (1978) discusses fishing communities. They have two adaptive strategies of diversification and intensification in order to react to changes in the fish stocks near the community. However, a strong central government usurped the power of problem definition by marginalizing local institutions and therefore bounded potential solutions. In this case, the boundaries excluded traditional individual adaptation mechanisms. This case exemplifies how a more traditional policy analysis missed existing solutions within the community and actually made the problem of fisheries management worse.
Institutional learning is important because in any complex adaptive system there will be surprises, especially since people respond to situations differently depending on their worldview (Kemp-Benedict, Bharwani et al. 2010). These surprises, however, are not a failure of the system – instead, they are necessary to provide signals for learning. Furthermore, since humans do not mechanistically self-organize or adapt, institutions provide the venue for that adaptation to occur (Berkes and Turner 2006). Gunderson and Carpenter (2006) elaborate on these concepts, describing two types of social changes necessary to maintain resilience – adaptability and transformability. Adaptability here refers to the capacity of the social components of a system to manage resilience and transformability refers to the capacity to create a fundamentally different system configuration when necessary. These changes require the ability to learn in three ways: a) incremental, which occurs as policies are implemented and evaluated, b) episodic, which occurs after environmental crisis or clear policy failure, and c) transformational, which attends to cross-scale surprises and accounts for the origin of novelty. They state “transformational learning is fostered by open social networks that link ecosystem understanding across social and ecological scales”, which is facilitated by institutions.
Institutions then account for linkages between social and environmental systems and provide the capability to learn and adapt. These interactions are not unidirectional, however – learning is constant and complex. For example, lessons from ecological studies that view ecosystems as dynamic instead of moving toward an equilibrium have taught policymakers that static policy will not be effective (McCay 1978). The feedback loop continues, however, as laws and rules are often influenced by cultural practices and moral norms but may also shape people’s idea of what is acceptable (Baland and Platteau 1996). Rules and community interact with one another to form human behavior, but Durrenberger and Palsson conclude from their fishing case that “due to the complex feedback loops in modern fishing and the nature of decision making, technocratic rationalization is pointless beyond a certain limit” (McCay 1987, p.389). Laws, rules, and community norms all shape the ecosystem as well, completing the loop of feedbacks that intimately connects humans and their ecosystem (Smith, Zhang et al. 2008).
The increased complexity of institutional analysis makes apparent the need for new methods for policy analysis. Document review of formal rules is still important, but it is now only a part of the picture. Here I will profile a few methods that have become particularly helpful to modern policy analysis pulled from other disciplines: a) network analysis (Janssen, Bodin et al. 2006), b) behavior and decision analysis (Kemp-Benedict, Bharwani et al. 2010), and c) cross-site comparisons (Poteete, Janssen et al. 2010). However, there is still a need to better bring together natural and social science methods as “no current or envisioned biophysical model can explain the vicissitudes of human will” (Kemp-Benedict, Bharwani et al. 2010).
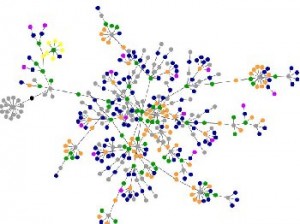
Network analysis and the more formal actor-network theory has much to offer in elucidating linkages in a system. Through network analysis, Janssen et al (2006) describe three types of socioecological system: ecosystems connected by people through flows of information and materials, ecosystems disconnected and fragmented by the action of people, and artificial ecosystems created by people. They suggest density, reachability, and centrality as metrics that may be used to indicate the health of socioecological systems. Density refers to the level of connectedness between actors, reachability refers to the number of links between actors, and centrality refers to the existence of well-connected actors that may have high levels of influence. The authors conclude “for the governance of ecological networks it is important to maintain the reachability of the system, for example, by conserving ecological nodes with high centrality”. Although this is only one example, it provides a compelling case for the utility of network analysis in policy analysis.
Modern policy analysis does not assume a certain type of behavior pattern for the actors in a system like the rational choice model, so additional methods are needed to empirically determine decision patterns. There is now an entire discipline of behavioral economics devoted to such a goal, a few of which have been tested in policy applications. The first is an expanded game theory with new types of games, repeated interactions, and new types of decision rules. Decisions can accurately be modeled, as evidenced by a behavioral model of rice farmers irrigating in Bali (Lansing 2006) who balanced water quantity and threat of pest outbreaks. Lansing describes his game theoretic model: “decisions about cooperation are influenced by the relative magnitude of the two relevant ecological factors, and these in turn are affected by where one’s fields are located. This creates a coupled system in which the players’ decisions influence local ecological conditions, which in turn affect the payoff for cooperating the next planting season” (80). The model not only predicted visible cropping patterns in Bali today, but exactly modeled what occurred during times of governance shifts following international agriculture pressures.
The other method successfully borrowed from behavioral economics is the decision tree. Kemp-Benedict et al (2010) used social data produced through knowledge elicitation tools with physical data produced from hydrological monitoring to make a decision tree incorporating mixed types of knowledge. The resultant tree was used as a “boundary tool” to provide a common language with which to discuss potential conservation interventions. Using such a tree can give insight into what possible outcomes will occur even though “gaining information about specific benefits and costs perceived by users at the time of collective-action decisions is, however, next to impossible” (Basurto and Ostrom 2009). Decision trees incorporate the costs and benefits without needing to explicitly quantify them, aiding in understanding decision patterns given what information is available.
The final method originates within anthropology’s cross-cultural comparisons and uses a consistent framework to compare across sites managing natural resources. Perhaps the most well-known of these studies is the International Forestry Resources and Institutions (IFRI) program started by Ostrom and colleagues nearly twenty years ago. They use the IAD framework to collect consistent data across sites. Early explorations with this program were critical to Ostrom’s (1990) development of the design principles for successful commons management. After careful, quantitative analysis of many cases, she was able to identify common traits among successful cases, noting that these are correlated design principles (not specific rules) that led to success.
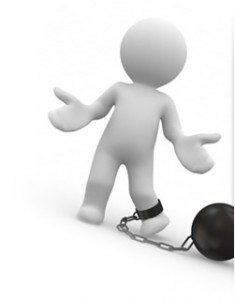
One final addition to the policy analysis toolbox added by the focus on institutions is the ability to use individual policies as experiments. Institutional learning includes the ability to change or remove policies that are not effective, an ideal that has not yet been realized within the governments of many large nations with leadership in international policy. Adaptive management emerges from many theoretical linkages between human and natural systems, from complex adaptive systems (Lansing 2003) to Gunderson and Holling’s (2002)complex adaptive cycle (another framework for linking social and natural systems), and finally the feedback loops from evaluation to exogenous variables in the IAD framework. Jentoft (2007) concludes “our ability to play with alternative images will to a great extent determine our ability to change, improve and innovate, since it is essential to institutional learning”. He advocates “playful, innovative management” with the ability to experiment with and end policies when necessary. He offers five new ways to treat concepts that have a history of slowing down institutional change: goals as hypotheses, intuition as real, hypocrisy as a transition, memory as an enemy, and experience as theory.
The next step in modern policy analysis will be to decide on the evaluative criteria in order to participate in adaptive management and policy experimentation. Berkes (2008) notes, referring to a couple of modern management challenges “in both the science of environmental toxicology and climate change, there is a sense that the use of a few indicators, no matter how well chosen, may be inadequate in capturing complexity” (pg. 202). A common metric from the business community is the concept of the ‘triple bottom line’ including environmental, economic, and social metrics. However, there is still much debate as to how to specifically measure variables from each of these categories and how to weight each category.